Visual Revolution: How AI is Reshaping Perception through Computer Vision
- Camgian Media Relations
- May 25, 2023
- 2 min read
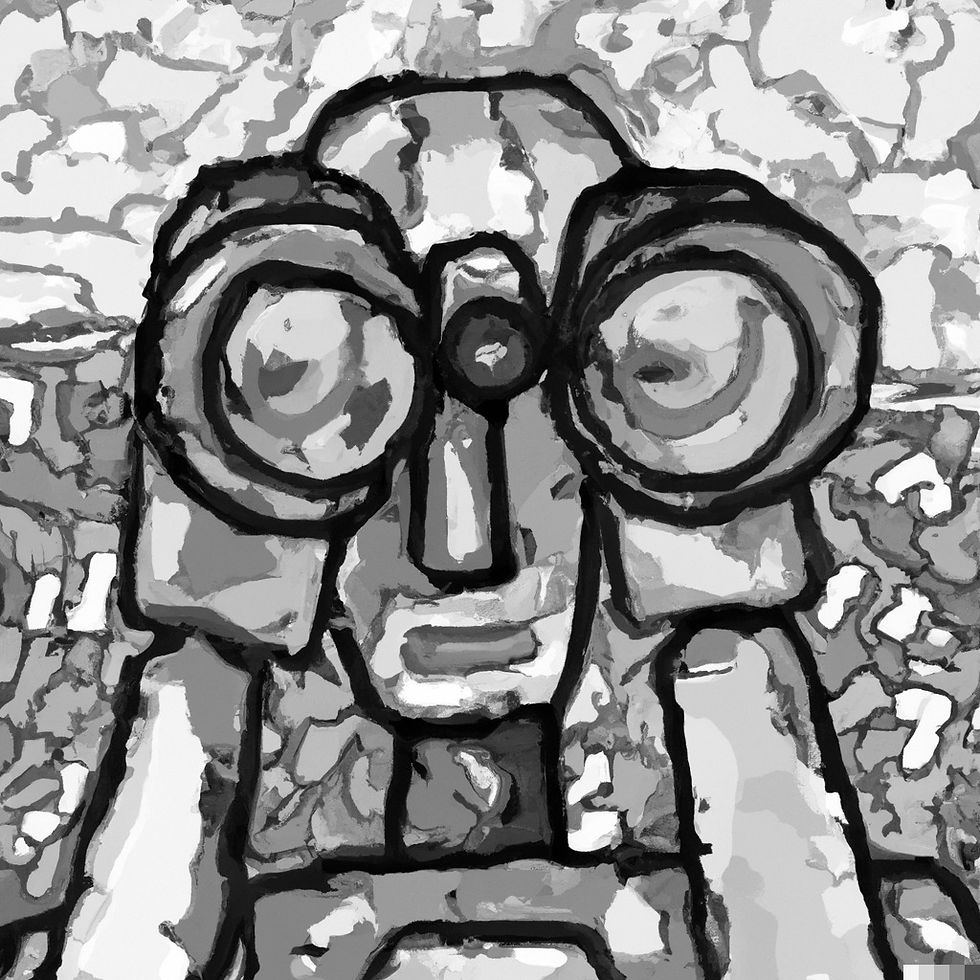
Computer vision, a branch of artificial intelligence, is revolutionizing the way machines perceive and interpret the world through visual information. By leveraging deep learning algorithms, machines can understand images and videos, surpassing human capabilities in tasks like object detection and image classification.
The applications of computer vision span across several diverse industries. In healthcare, it aids in early disease detection and surgical procedures, potentially saving lives and improving patient outcomes. In retail, it enables personalized shopping experiences and automates inventory management. Moreover, computer vision plays a vital role in autonomous vehicles and robotics, empowering machines to perceive and interact with the environment to make real-time decisions.
Although neural networks were first proposed in the 1940’s, the hardware at the time was lagging these advancements and proved they were not feasible for real-world applications. However, with the introduction of powerful GPUs and parallel computing, researchers were able to train deep neural networks on large-scale data, proving their practicality and effectiveness for real-world vision applications. Now, these networks have become an important part of everyday life and can be found everywhere from industrial servers to the compact mobile device in your pocket.
Advancements in computer vision are driven by readily available large-scale datasets, improved computing power, and breakthroughs in deep learning (DL) algorithms such as Convolutional Neural Networks (CNNs) and, more recently, Vision Transformers (ViTs). These networks learn complex visual representations, enabling machines to generate a wide range of information in image or video data, depending on the task they were trained for. Training a network from scratch is a tedious task that requires access to powerful hardware, large-scale data, and time. Fortunately, pre-trained models are widely available and transfer learning can be utilized to quickly fine-tune network parameters for a task using commercially available hardware.
Many different technologies fall under the computer vision umbrella. End-to-end object detection and recognition models are trained to localize and provide a class label for objects in a scene. DL-based tracking algorithms leverage the feature-learning capabilities to reliably follow a moving object in video data. Image segmentation decomposes a scene into pixel-level class labels to provide distinct separation between objects. Temporal analysis of visual information provides a means to perform activity recognition and anomalous event detection. 3D reconstruction involves generating the 3D environment from 2D input using algorithms such as SLAM, stereovision, and monocular depth estimation. These branches often overlap and when combined can provide a deep understanding of the environment and allow machines to perform higher-level functions in the world. In conclusion, computer vision transforms how machines see and interact with the world. Its applications offer increased efficiency and automation, fueling innovation in diverse industries. As technology continues to progress, computer vision opens new frontiers and paves the way for a future where machines possess unparalleled perception and understanding.
Commentaires